In search for the next generation of DNN models that mimic the concept-based paradigm
Turing defined intelligent machines as those that may indistinguishably imitate their counter human-agents in lingual communications. This game of imitation was the challenge taken by the field of artificial intelligence over the previous seven decades. Such a challenge is currently considered, by many, as a historical test that AI managed to remarkably supersede in lingual, visual, and creative tasks. Nonetheless, reality declines these proclamations. For example, we have been promised full self-driving cars by 2020, but that is not on the visible horizon; Image recognition is susceptible to adversarial examples; Chatbots are of limited capabilities. Therefore, there are Irreversible limitations inherent in DL that are keeping DL from reaching the enthusing milestones we once sat.
On the other hand, another school of data scientists calls for reasoning and causality to compensate for the shortcomings of the DL paradigm. It presumes reasoning and causality as the best way for mimicking the human agents. Nonetheless, reasoning can’t model the different faculties of the mind. For example, analogy making/understanding, which is the main gist of art and a main substrate of the mind’s processes, can’t be explicated using the tools of reasoning
In brevity, both the DL and reasoning approaches are applied over segments of knowledge that are bounded by the correlated spaces of the inputs and outputs. They act over the syntax of the modeled domain, and they lack any connectivity beyond the encoded syntax of the problem under analysis. The semantic connectivity that relates these disparate segments altogether is missing.
The post proposes a dramatically different trajectory. The post presumes that an intelligent machine has to possess the capacity of philosophization. An intelligent agent is a philosophizing-capable machine.
In brevity, both the DL and reasoning approaches are applied over segments of knowledge that are bounded by the correlated spaces of the inputs and outputs. They act over the syntax of the modeled domain, and they lack any connectivity beyond the encoded syntax of the problem under analysis. The semantic connectivity that relates these disparate segments altogether is missing.
Nonetheless, for such a philosophical discourse to be established, there must be a backing knowledge base (KB) of reality. Such knowledgebase has to sustain analogy making/understanding. In fact, philosophization is a mere procedure practiced over such KB, and consequently, establishing a philosophizing-capable machine means establishing the specified KB .
In the post, I’m excerpting the findings of my Ph.D. research for establishing a creative machine that augments an architectural/urban design process. The research structures a knowledge base of conceptualizations. Each concept found in natural language is unfolded by its own conceptual space. Over seven publications, I grounded a tri-perspectival ontology of urban constructs. These three perspectives are named Rational, Systemic, and Visual, and they are proved to be the minimal contrasting perspectives, and they were substantiated for their sufficiency in constructing the needed knowledge base of conceptions. By the way, I got a big “A” from a prestigious international committee, and therefore, the proposed conceptualization has tangibility in the field of design creativity.
The three perspectives compete in shaping the conceptual spaces of any concept. These conceptual spaces are the fundamental units that monolithically span all the faculties of the mind (including art), analogously similar to the atoms of the physical reality. Such a self-regulatory competitive behavior promises the computational-ability of establishing the fundamental atomic conceptions of all the basic concepts found in natural language. These fundamental conceptual spaces are infinitely composable by the machine for understanding/producing new concepts.
The three perspectives compete in shaping the conceptual spaces of any concept. These conceptual spaces are the fundamentConsequently, the proposed conceptual spaces have to explicate the various facets, properties, and realities of any fundamental concepts. Structuring these fundamental concepts as a whole manifests the proposed KB. A philosophizing discourse discloses any of these different faces at a time, Much like in quantum mechanics, all the various states are in a superposition until observed. In Equation (2) [later in the post], I explicated how the concept-based paradigm implies a novel different computational approach. In fact, concept-based ML is the best fit for the revolutionary quantum computing.al units that monolithically span all the faculties of the mind (including art), analogously similar to the atoms of the physical reality. Such a self-regulatory competitive behavior promises the computational-ability of establishing the fundamental atomic conceptions of all the basic concepts found in natural language. These fundamental conceptual spaces are infinitely composable by the machine for understanding/producing new concepts.
Such a computational model infuses general and narrow intelligence as a mere concept-based intelligence. As much as it infuses the physical materiality and the conceptual immateriality, or the syntax and semantics, under a singular concept-based reality.
In brevity, the concept-based paradigm is the most general; it possesses the capacity of modeling both worlds as a single realm.
Based on that, In the universe of the concept-based paradigm, only one of two types of projects may manifest:
1. Projects that mimic certain qualitative/faceted variants of the fundamental concepts or their compositions.
2. Projects that supplement the knowledge base (KB) needed by an AGI agent. Such KB should disclose the variants of all the concepts in a coherent whole, and it has to additionally sustain analogy making/understanding.
To support the point of view of the post (POV) that the concept-based paradigm and the philosophizing-capable machines are the trajectories for the futuristic development of the field, I have included three prevalent exemplary case studies along with my research findings. The three selected cases are as the following:
- GLOM and DreamCoder, as a support to the first project types of the concept-based mimicry assumption.
- SingularityNet/OpenCog, as a support to the second project types of the supplementary of a KB that sustains intelligence.
The post presumes its clarity in disclosing its POV by proceeding in a definitive path. It starts by differentiating between the DL and concept-based paradigms before ruling out reasoning (as contemporarily defined) as a viable tool for AI development. The post then encloses a brief of my research along with the three exemplary cases before wrapping up their contribution is supporting the post’s POV. Based on that, the post structures according to the following table of contents.
Table of contents:
As a final note, the post may be a bit longer than what it should regularly be, but I preferred structuring the subject in a single post rather than dividing it over several posts. After all, it took me a longer time trying to shorten the post than it took me writing it. The reader may skip to the wrap-up section after the introduction and before the sequential navigation of the whole post if needed.
I have compiled the criteria that distinctively distance the two paradigms from each other in Table (1). I may summarize the most unique qualities of the two paradigms according to the following:
The deep learning paradigm:
For the sake of simplicity, I would consider only the DL supervised learning as a representative of DL. DL excels in correlating the related patterns between the space of the inputs to the space of outputs. The input/output spaces are represented by Tensors. The DL model is merely a mathematical function that agglomerates smaller parametrized functions, and the parameters of these compositing smaller functions are optimized during the training process using backpropagation. The goal of the trained DL model is to predict the classification (in case the output space is discrete) or regression (when the output space is continuous) of the recognized patterns of the input space’s tensors. To optimize the DL parameters, sample training data points are needed. Optimizing the parameters of the DL function means that its hypersurface goes through all these training sample points. Nonetheless, doing so yields what is known as overfitting. On the other hand, smoothing the hypersurface means that it may generalize beyond the sample points, and this may be partly done by eliminating some of the sample points as outliers and averaging the least squared distance between the rest. The duality between overfitting-generalization is a persistent challenge that affects the quality of the functionally approximated predictions, and the cross-validation technique is a common practice to counteract this challenge.
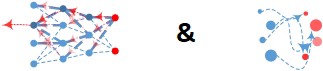
Consequently, DL always acts interpolatively on segments of knowledge that are depicted by the input-output spaces, and that is the undeniable general actuality of deep learning. Meaning that it doesn’t matter what is your DL model of choice, the previous description applies. For example, if your model of choice is the multi-perceptron layers for classification, a convolutional deep model for grasping the local patterns of the input space, temporality grasping models like RNNs, or contextually attentive models like LSTMs and transformers, there have to be predefined spaces of input’s features and output’s classes.
Although it is proved that DL is a universal function approximator, meaning that if there are a magnitude of segments of knowledge involved in the system under analysis, DL models are still capable of modeling such a system, and whence may theoretically model knowledge in its entirety, by the end of the post at the “to wrap up” section, it would be quite apparent that such a DL’s segmental representation of knowledge is unrectifiable.
Accordingly, and although the reader may find a wider range of limitations/capabilities of the DL paradigm in Table (1), I think that the greatest limitation of the DL model is its containment in its input/output spaces. There is no whatsoever connectivity to any context laying beyond the boundaries overlaid by the tensors representing these two spaces. Consequently, No matter how massive data is collected and preprocessed or how powerful computation is employed, this limitation is irreversibly rectifiable. Meaning that this limitation is inherent in the deep learning paradigm, and that is why we are in desperate need of new approaches, which I propose to be a hybridized manifestations of the concept-based model, which (in contrast) is all about the connectivity and structurality, as disclosed in the following section.
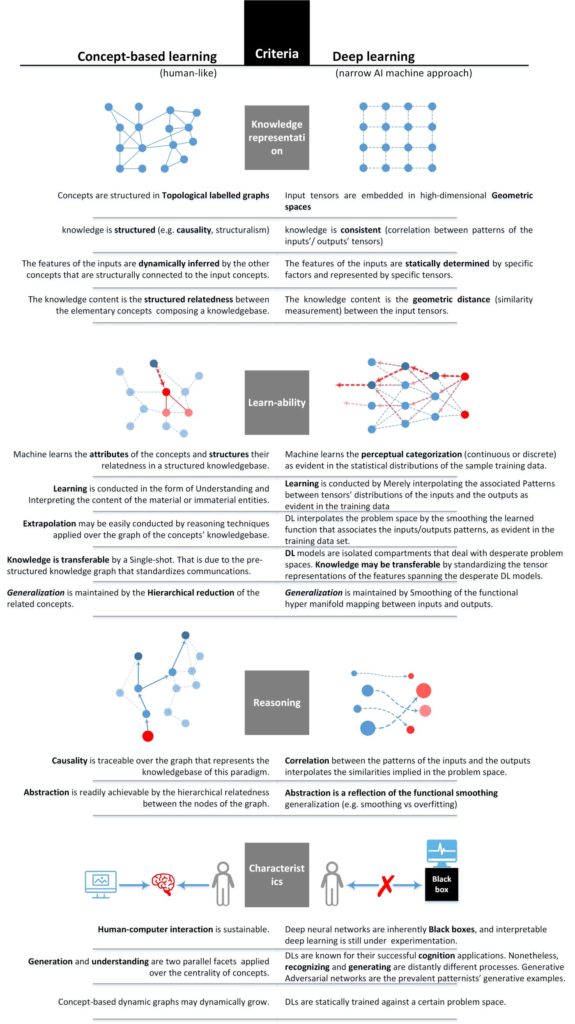
Table 1 a categorical comparison between the concept-based and the deep learning paradigms. (Table by Author)
The concept-based paradigm:
This paradigm revolves around the centrality of concepts. Meaning that the concept-based paradigm manifests by structuring the elementary units of concepts. For example, a single concept like “walking” is comprehensible when related to other concepts. Therefore, the different facets of this exemplar concept (such as, the act of walking, the requirements for conducting this action, the implications of walking, amongst plenty of other facets of such a concept) would collectively structure what is known to be the concept of “walking”. In fact, a precursor, and the founding movement, of modern philosophy is a movement known as “structuralism”. According to structuralism, a concept is the basis of human epistemological and sensual experiences, and it posses its meaning when related to other concepts. Such structurality is currently best represented by graphs and it entails the solidarity of either understanding or generation to be equally practiced over the backing knowledgebase of concepts. For example, natural language understanding may go through the same process as that of natural language generation. Additionally, this paradigm implies the one-shot learnability, one-shot knowledge transferability, machine-human communication over the common knowledgebase of concepts, explorability/interpretability, dynamicity, and the potentials of extrapolative generalization.
Although there are certain confusing similarities between the concept-based paradigm and the historical GOFAI (Good Old Fashioned Artificial Intelligence, alternatively known as expert systems), these two approaches are quite dissimilar from each other. The GOFAI is a procedural graph populated by an expert human agent, while the concept-based graph declaratively structures a knowledge base about the elementary basic concepts. Such a graph consumes the fundamental knowledge base needed for an artificial agent to harvest intelligence, which may be analogously related to the commonsense of the human agents.
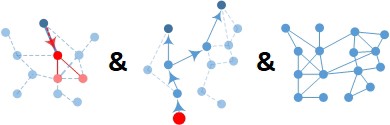
The differentiating qualities listed in Table (1) may satisfactorily span several books of careful analysis. Nonetheless, in the coming sections of the post, I would concentrate on the single quality of connectivity. Doing so would not only convey a concise message out of this post, but it mainly reflects a belief that this is the main quality that may causatively originate all the other stemmed qualities listed in Table (1).
Connectivity is always looked at as the substrate that substantiates reasoning mechanics. If there is one widely known school that may currently compete with the patternist approach of deep learning that would be causality and reason, which are the tools for proclaiming understanding rather than the sketching correlations by deep learning. Consequently, in the coming section, I would briefly touch on what is intrinsically meant by reasoning in preparation for accentuating on its deficiency as a sole contributor of the phenomenon of the human mind. For example, analogy making/understanding, which is the foundation of art, is not explicable by reasoning.
2. What is “reasoning”?
Reasoning may be defined as the process of explicating new knowledge based on what a human agent may already know. In other words, it is about inferring valid conclusions out of any given premises. Three forms of reasoning are widely known, and they are deductive, inductive, and abductive reasoning mechanics. Deductive reasoning is a direct (automatic) inferential mechanic, e.g., the two premises of “all dogs have four legs” and “I have a dog” then “my dog has four legs” is a deductive inference, while the other two reasoning mechanics deduce plausible conclusions that either generalize or abstractly simplify the premises into a best uncertain guess. Nonetheless, Reasoning mechanics are applied over structured representations of the premises and conclusion, and the two widely known knowledge representations are the Symbolic First-Order Logic (or higher) and the Probabilistic Bayesian Networks; both of these two knowledge representations may represent the premises and the conclusions by parsing them as a graph. Consequently, reasoning has to be conducted over graphs, similar to the concept-based paradigm’s knowledge representation.
Although there is a strong belief in the community of data scientists that reasoning may be our way out to compensate for the shortcoming of the DL paradigm, solid empirical and philosophical opinions argue against that. For example, Mark Bishop advocates the deficiency of reasoning as a tool for AGI, and his arguments are plainly stated in one of his latest papers titled “Artificial Intelligence is stupid and causal reasoning won’t fix it” [2].
The post may be in line with Bishop‘s argument, but in a fairly different attitude. My arguments may be attributed to the following:
- Reasoning mechanics are merely practiced over the Syntactic formations using the tool of logic algebra. They are not practiced over the semantic content of the premises or the conclusions. For example, the premise of “John walks every day to work bare feet on the water with his nephew Joseph” would syntactically imply that “Joseph walks bare feet on the water”. Nonetheless, I and you are pretty sure that neither John nor Joseph (or anyone else) may walk bare feet on the water. The evidence here is in the Content not in the syntax itself. Consequently, syntactic reasoning is a mere pale replica of the DL paradigm, and it consequently still holds all the limitations of DL. Therefore, for reasoning mechanics to be worthy of the ambition of the futuristic development of the field, it is semantic reasoning that is needed. Nonetheless, semantic reasoning requires a knowledgebase about the world, (for instance, such a knowledgebase should contain the metaphor I used of the inability of walking bare feet on the water).
- Reasoning alone may fail to elucidate different faculties of the mind. For example, emotional intelligence is considered the main fuel of human intelligence, and it supersedes in its influence any IQ tests of intelligence. For instance, Art is not explicable by the tool of reasoning. Art is debated by prominent philosophers that it survives on analogy. For artists, art is generated by Analogy making, while for perceivers, art is sensed by analogy disentanglement. you may be amazed that these results experimentally resonate with findings of the field of neuroaesthetics. Neuroaesthetisists empirically study the human brain’s behaviors associated with aesthetical phenomena. Photoimaging the frontal loop assured specific places that fire in correlation with artworks, and they are located near decision-making regions in the frontal loop. These results are universally consistent amongst humans, and they substantiate the assumption that aesthetics is a kind of reasoning that has to be analogical.
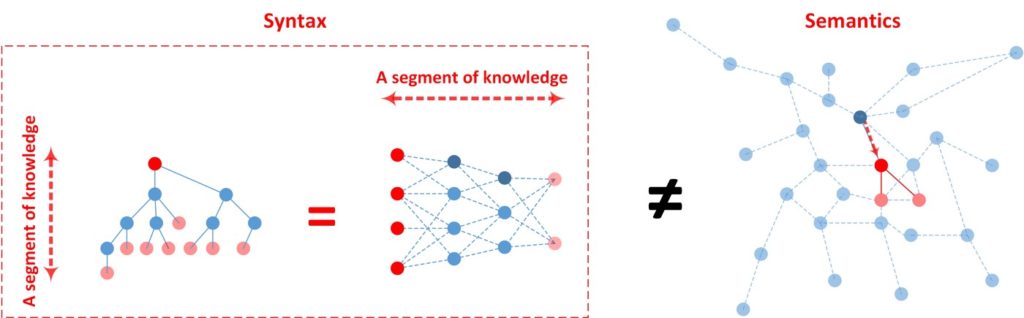
Reasoning is a pale replica of DL; both of them act on bounded segments of syntactic knowledge, although they may misleadingly appear as two different approaches. On the other hand, semantic-based processing that is backed by a knowledgebase of reality entails a different computational approach (see Equation 2). In such a computational model, inputs and outputs are themselves subjected to deliberation; computation has a searching space usable for accentuating on different possibilities of the input/output variations (Image by Author).
We have to note here that syntactic versus semantic reasoning is not a trivial issue, but rather a foundational one that distinctively distances the two main forms of knowledge, which are concrete sciences (syntactic formations) and philosophy (semantic content).
What I’m trying to say here is that art is practiced/experienced by analogy, and consequently, it builds on substrates similar to that of human reasoning, not the computational version. In other words, there is a monolithic tool of analysis that equally underlies art and reasoning, and that is analogy. The thing is that analogy has to be practiced over a knowledge base of reality; Some may call it common knowledge about the world or even commonsense.
Based on that, I may differentiate between art and inferential reasoning by the following:
- Inferential Reasoning is Intentionally attended over such a knowledge base of reality. Therefore, processes like thinking, evaluating, deciding, etc. are practiced by controlled involvement with such a knowledge base.
- Art is an unintentional attended involvement with an entity, which is either material or immaterial. Such involvement shakes our beliefs of the world, which is represented by the knowledge base of reality, and it introduces novel unvisited realities about the entities in attention. That is why art is always associated with creativity, novelty, surprise, and imaginability.
In the coming two sections I’m sharing a brief of my Ph.D. research’s results for constructing such a knowledge base. The proposed knowledge base builds over the fundamental unit of concepts. Therefore (metaphorically speaking), in the physical realm, it is the atoms, while in the mental world, it is the concepts that agglomerate into the versatile/complex realities we wetness by our sensorial systems and go through by our phenomenological experiences.
3. Analogy as a main player of reasoning/creativity (my research’s contribution):
The marvel of establishing a single concept-based tool of analysis that spans both the concrete and soft sciences (science and philosophy) may exceed our expectations. The possibility of having a single approach to cover the two intentional/emotional intelligence, which may seem quite different, is based on the assumption that two common actualities equivalently substantiate both of them, and these two actualities are differentiation and structuralism. The reasons for assuming differentiability and structurality as the basic common traits of human intentional/emotional intelligence may be clarified by the following:
- To epistemologically know requires the capacity to differentiate between the composing entities, while to be experientially involved mandates the unintentional distinguishability between the interacting entities. The capacities of differentiability/distinguishability are two faces of the same coin of differentiation.
- The distinct different/distinguishable entities have to structure into what is known as the knowledgebase of related distinct entities, which may be alternatively known as structuralism.
Consequently, the secret key here is structuring the variations in a bigger whole that may be considered fundamental for the processes of understanding/experiencing. As simple as it may seem, as powerful as we may imagine. For example, probability distributions, which are the basis of the probability theory and statistics, or variance, which is what calculus is all about, are the mere applications of observing and analyzing variations of a population or a dynamic system. Additionally, In cognition and the science of mind, structuring the variant perspectives may be recognized as semantic networks, which is a more common term used by computer/data scientists.
The two main questions that immediately follow are: structuring the variations of what, and how would those variations be structured?
As for the what to structure, it is the concepts. Concepts are believed to be the atoms that substantiate all the faculties of the mind.
As for the how to structure the concepts into a bigger whole, the only way is to find commonalities between all the concepts. Finding the most fundamental formative forces that make each concept distinguishable, different, distinct, or simply unique, is the ontological question that has to be investigated.
Nonetheless, we need to keep in mind that if there are tangible traces to the world of concepts, then that would surely be natural language. Natural language is the material tool for scientifically analyzing the immaterial world of concepts. Nonetheless, urban constructs (the built environments) that humanity constructed over the years are surely another material trace of the world of mind, and that emphasizes my research’s findings as an indispensable supplementary tool of analysis along with natural language processing.nalities between all the concepts. Finding the most fundamental formative forces that make each concept distinguishable, different, distinct, or simply unique, is the ontological question that has to be investigated.
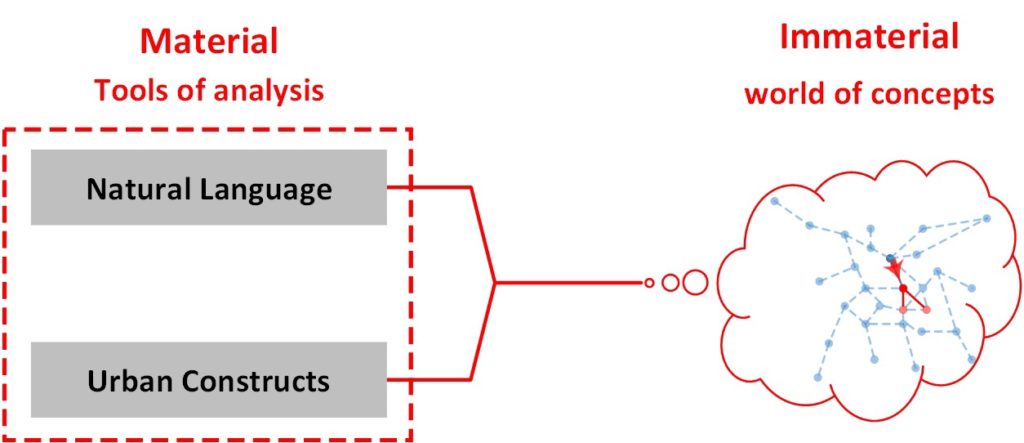
natural language and urban constructs as the two known material traces for studying the immaterial world of concepts (Image by Author).
Therefore, the challenge here is how to find such an ontology, and my Ph.D. research was about coming up with such an ontology. To do so, I studied all the known urban constructs, the known theories, and movements from history to the current time. I came up with three perspectives that I named rational, systemic, and visual, and these perspectives were argued during my research for their sufficiency in constructing the needed knowledge base of conceptions, or in other words, constructing the variants known of any concept into a coherent whole, and I got “A” as a consequence (see Figure 2).
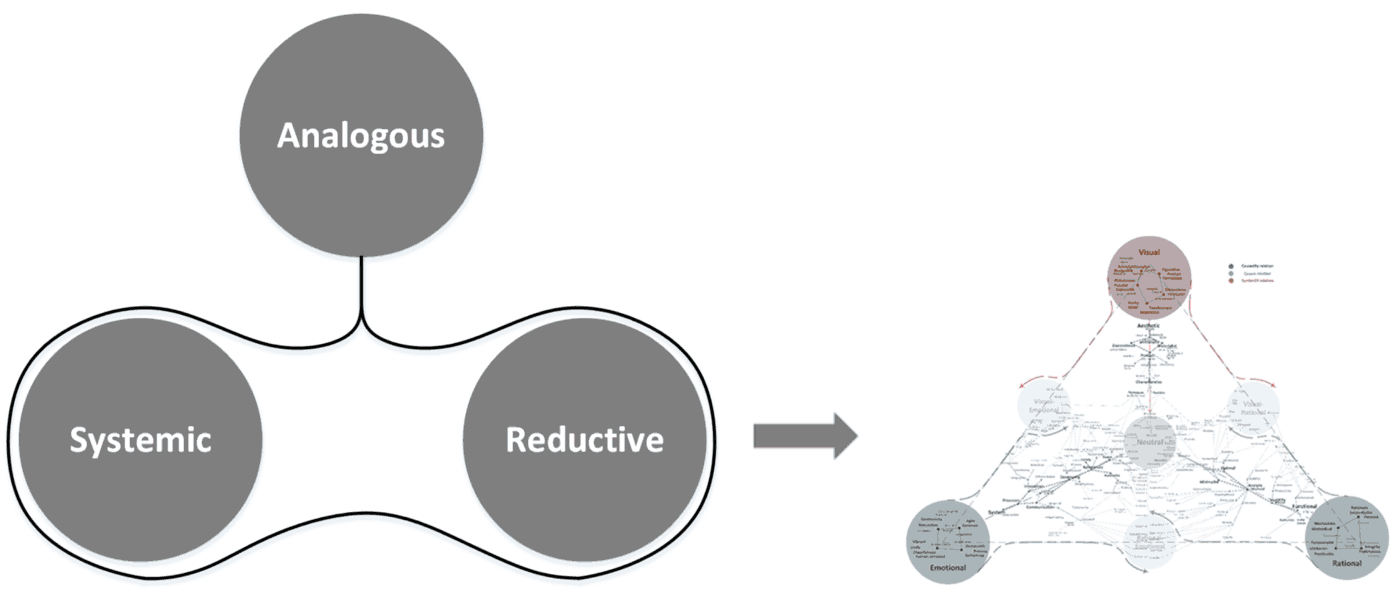
Figure 2 my research grounds the proposal that three perspectives would sufficiently structure a conceptual space of any concept found in natural language. Such a conceptual space is dually embedded and graph-based represented (the right figure is an exemplar conceptual space of the concept of “functionality”). (Image by Author).
Although I’m planning to unfold my research over several posts, including exhibiting the constructed conception proposed by my research as the basis for a creative machine, I may concisely portray the three perspectives as the following:
- The rational perspective: is a reductive view of the universe. The whole is equivalent to the summation of its parts, and consequently, truthful ethicality, logic, usefulness, and productivity are amongst the values appreciated by this perspective.
- The systemic perspective: is a bottom-up perspective that is shaped by the procedural emergent interactions between its granular elements.
- The visual perspective: is the creative philosopher that narrates the analogies made by mixing the rational and systemic realities. This perspective is what a creative machine is all about, and its computational details are specified in Equation (2).
It is important to note that These three perspectives are infinitely definable because although they are used to expose the various facets of any concept, the defined concepts themselves cyclically extend the three perspectives’ definitions. The definition of the three perspectives and the knowledge base of concepts is self-regulated by computation with minimal human-side involvement.
Based on that, I would momentarily outline my research in the following few paragraphs and the coming section as well.
My Ph.D. project’s goal is to establish a creative machine that may augment human agents in creatively designing urban constructs. The main belief that I have had since the inception of my project, seven years ago, is that concepts are the central elements needed to unilaterally encompass the different forms of emotional, cognitive, and procedural intelligence. Additionally, they are the fundamental blocks that may explicate the indispensable role of art in establishing the phenomenon of the human mind. Based on that, I published a series of articles that substantiate the theorization and the implementation of my proposed model, e.g. seven chapters, conference papers, and journal articles, and if you are coming from a background similar to mine, you may find three of these articles [3, 4, 5] interesting to read. In these three papers, one of which is translated and republished in a major urban scientific journal, I introduced an ontology of urban constructs for constructing the conceptual spaces of the concepts.
Therefore, my research establishes a universal conceptual space that is argued, and to a high degree established, to substantiate emotional and intentional human intelligence. Each concept found in natural language manifests in a conceptual space of its own, and such a conceptual space is dually represented by a geometric space (embedded) and a graph-based representation (see Figure 2).
The agglomeration of these conceptual spaces over all the concepts found in natural language constructs a conceptualization that may be used as the basis for human-computer interaction over a creative design process (see Figure 3.3), and such fundamental conceptualization is composable for the creation of the infinitely complex new conceptions. Nonetheless, to construct the proposed conceptualization, I came up with a concise procedure that is illustrated in Figure (3).
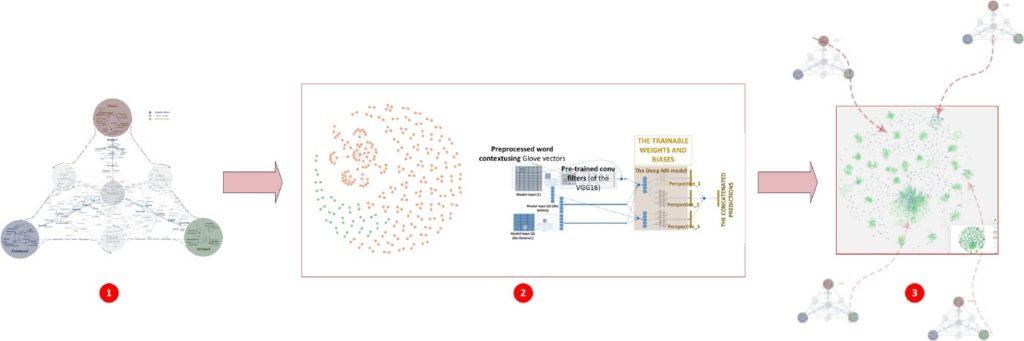
Figure 3 the conceptual space of the fundamental concepts. Several conceptual spaces (Figure 3.1) are used as a training dataset for a DNN model that is presented in (Figure 3.2). The trained DNN model then predicts the concept spaces of all the concepts of natural language, and whence these predicted concept spaces agglomerate into the proposed knowledgebase (Figure 3.3). The conceptual spaces of all the concepts are agglomerated in the backing graph database (NEO4J database manager) to construct the proposed conceptualization. (Image by Author)
The proposed conceptualization proposed by my Ph.D. is whence assumed to play an indispensable role in artificial general intelligent agents (AGI) and fusing narrow AI and AGI into a single concept-based approach, and its computational implications are illustrated in the following section.
4. The computational implications of my proposed tri-perspectival-based conceptualization:
It is worth a while to conclude the previous discussion of the three perspectival perceptions of the elementary units of concepts by procuring their computational implications.
The two systemic and rational perspectives maintain singular realities that may be described by the following equation:

Equation 1 the standard formula for the hard sciences (including data science). A model F() is structured and optimized for emulating The relatedness between the observed inputs/outputs of any system, and that applies perfectly for the systemic and rational perspectives of my Ph.D. research. (Image by Author)
Having a deeper look at this equation, we may find that Function (1) is the intrinsic reality of all concrete sciences, including the field of data science. In Equation (1), “In” and “Out” are the observed/measured data samples that are modeled by F(), and the parameters of F() [θ] are optimized so that F() models the phenomenon established by correlating “In” and “Out”. And in the current practice, such a function can model the rational structurality and the systemic dynamicity (that is mostly in the form of differential equations).
On the other hand, the visual perspective is the creative one that reinterprets these systemic and rational realities into new unvisited interpretations, and this is the model the post publicizes as the basis for the forthcoming development of the field. Equation (2) describes how the visual perspective’s interpretations depend on the knowledge base of the rational/systemic reality, as manifested in Figure (3.3). in Equation (2), the inputs and outputs are themselves reinterpretable by the machine. Consequently, there are no limitations of the predefined tensors of the inputs and the outputs, which is the case with contemporary narrow AI, but rather the machine possesses the capacity of understanding, thinking, and determining, which are practiced based on the machine’s preferences. This may be matched with computational free-well that should be ethically tamed by training the machine’s selectivity versus common human preferences and behaviors.
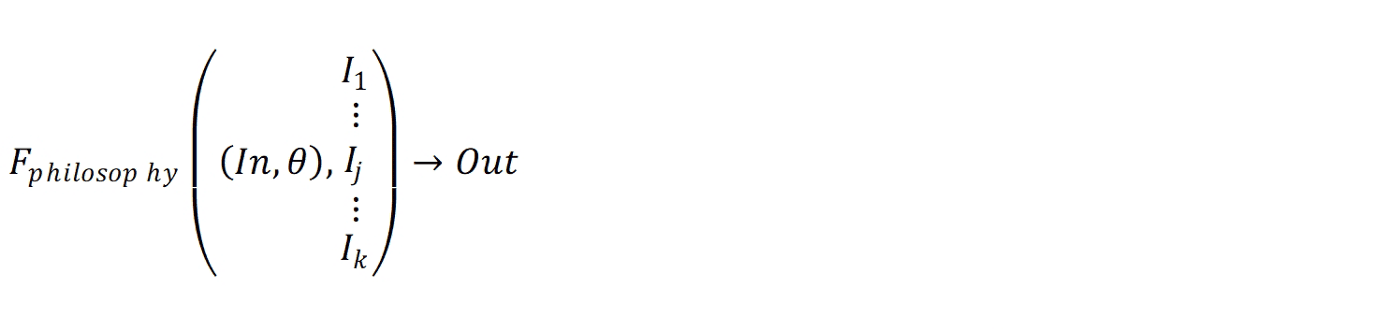
Equation 2 the formula presumed by the post for structuring a concept-based intelligent machine that maintains the capacity of philosophization. the machine is presumed to maintain a knowledgebase about reality (In), and it utilizes it to reinterpret the inputs and the outputs of Equation(1), as in the right side of the formula. (Image by Author)
5. Exemplary Three Case studies:
The qualitative comparison between the two paradigms is the post’s first step in hybridizing the two paradigms into a single realm that supersedes the limitations imposed by each. Although numerous cases support the post’s point of view, I have selected three recent, prevalent ones. These selected cases highlight certain qualities unfolded in Table(1), and they are as the following:
1. Hierarchical convolutional neural networks (GLOM) [6]:
(part-whole hierarchical neural network)
Geoffrey Hinton is one of the three leaders who shaped what we may currently recognize as the field of DL. Hinton’s 2012 paper [7]that applied Convolutional Neural Network (CNN) for the ImageNet challenge, in which their trained model (AlexNet) sat an unmatched benchmark for computer vision’s image recognition, which is still the state of the art model that is only superseded by other CNN variants, e.g., deeper CNNs and residual CNNs. In convolutional neural networks, kernels of fixed sizes (typically 2×2, 3×3, 4×4, etc.) are trained to recognize local patterns of the image’s pixels, and such mere input-output correlation belongs to the post’s first paradigm of deep learning, though it grasps the per-kernel localities.
Nonetheless, in his latest publication [6], Hinton took a dramatically different trajectory. In that publication, he proposes an experimental symbolic-like approach for image recognition by interpretation. By doing so, Hinton finally recognizes interpretation as the basis for cognition. In this new approach, each pixel of the image belongs to a hierarchy of part-to-whole conception. For example, a pixel may belong to an eye, which is part of a face, which is part of a person (or an animal or so), which is part of a place, which is part of a scene. Consequently, there must be an agreement between subpart-part-object-scene for interpreting each pixel in an image. Meaning that the agreement between subpart-part-object-scene is the key for constructing a graph that symbolically recognizes any image.
The takeaway points of this experimentation are that:
- Image recognition is implemented over a graph, and whence it is following the symbolic path of understanding objects rather than merely the functional association between pixel patterns of the inputs and classes of the outputs.
- A predetermined ontology of the objects-subobjects is a prerequisite for defining a DNN model of fixed size.
- It employs the DNN techniques for constructing an understanding, and whence it blurs the boundaries between specific-task and general intelligence by introducing understanding as the basis of cognition.
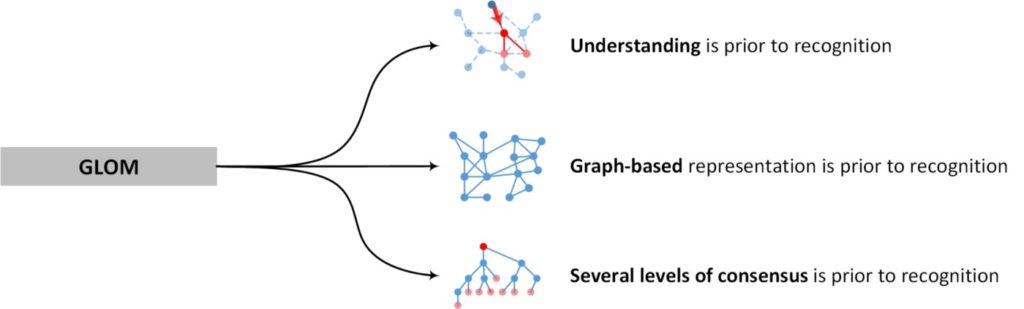
2. DreamCoder [8]:
The goal of DreamCoder is to be a symbolic problem solver by using lingual compositions. Meaning that there are basic concepts and the language that arises by compositing new concepts out of these basic concepts would enable computation to be more human-like for creative and problem-solving tasks. The thing is that DreamCoder gets better at learning different compositions (new concepts) and then utilizes these newly learned languages scalabily using neural networks.
The takeaway points are as the following:
- DreamCoder acts on eight specific basic conceptual domains, which are “list processing”, “text editing”, “Regexes”, “Logo Graphics”, “Block Towers”, “Symbolic regression”, “Recursive Programming”, and “Physical Laws”. This may be considered as an implicit ontology DreamCoder utilizes throughout its processes.
- DreamCoder employs a “wake-sleep” learning algorithm. In the sleep mode, the algorithm fictionizes challenges to grow its warehouse of composed concepts, while in the wake mode, it uses its learned knowledge base of concepts for solving problems by symbolically composing the already learned concepts.
- It utilizes DL narrow AI techniques (pairing specific inputs-outputs) for the wake/sleep algorithms. Meaning that it employs a deep learning paradigm for mimicking traits of the concept-based paradigm.
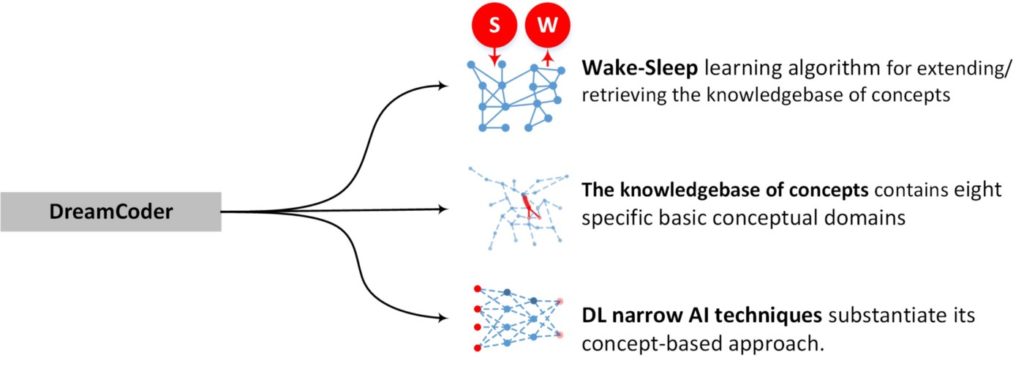
3. SingularityNET [12,13]:
Dr. Ben Goertzel is an influential figure in the field of AGI. In fact, the reader may grasp a good idea about what is AGI, what is expected out of it, and how to procure an AGI system by reading Dr. Goertzel‘s latest paper [9]. The paper is a glorification of his earlier books and papers. Dr. Goertzel is widely known for his projects of OpenCog, SingularityNET, and the superstar Sophia (the robot) that is an application of his latest project (SingularityNET). Although SingularityNET is the contemporary well-publicized project, and some of us may have already monetized a specific task trained model in that Global AI Marketplace, OpenCog (which is an older project) is a significant contribution to the field of AGI. In fact, OpenCog, and all the team working on it, are now an integrative part of SingularityNET. Based on that, I would summarize both of these projects as the following:
a. OpenCog manifests a declarative knowledge base as the basis of any AGI agent [10,11]. Such a declarative knowledge base is known as Atomspace, and it is represented by a metagraph. The atomspace builds upon fundamental atoms, and it is expected to be there more than 64000 atoms consuming the atomspace. Other forms of knowledge and faculties of the mind are laid over the atomspace.
b. SingularityNET is a global AI marketplace [12,13]. It is mainly a decentralized protocol that organizes the communication between smaller trained models that synergically interact for creating a bigger smarter systems. Therefore, a model trained to perform a specific task may be offered on the SingularityNET platform for other larger smarter models to structure as one of its components. Such a larger model may itself be composed as a part of even a larger smarter model, and Sophia, the robot, is expected to perform as an AGI agent in a similar way.
The takeaway points of these two projects are as the following:
- The atomic structure of the declarative knowledge base of OpenCog is proposed as the foundation of any AGI agent.
- The atomspace is represented by a metagraph.
- Narrow DL techniques substantiate the structuring of the atomsapce and its coupled dynamics.
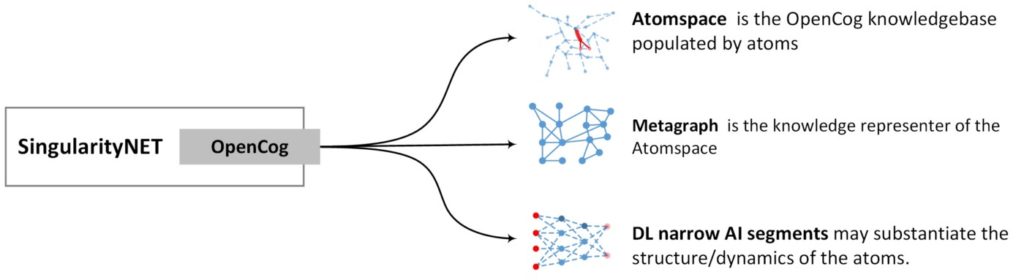
6. To wrap up:
The reader may be amazed, after all, that deep learning is known as connectionism; metaphorical to the neurological connections between the neurological cells, and such connectivity mimics what is believed to be a brain that performs as a computational machine. Deep learning abstracts this biological connectivity using mathematical structures, and although it did well in establishing universal functional approximators, it still lacks the main gist of connectivity, which is otherwise quite prevalent and is the hallmark of the concept-based paradigm. Indeed, the notion of connectivity and extension is believed to be, although quite simple, of high influence in explicating the human mind. Conceptual connectivity is debated throughout the post for its fundamental role in mimicking the two intertwined realities that demarcate the human mind’s activities, which are either intentional or emotional. That is why the concept-based paradigm has much better chances to be referred to as “connectionism” than the DL’s neurological connectivity ought to be.
Nonetheless, connectivity on its own is not sufficient for explicating the human mind, as a matter of fact, other projects tended to rely merely on the notion of connectivity but achieved less prevalent results. That is why my project dictates that such connectivity should manifest in the form of structuring the variants of the basic concepts, and based on these foundational concepts, concepts of infinite complexity may emerge. And by intuition, a knowledge base of conceptions, by its definition, has to maintain all the variants known for any concept. My research proceeded in that direction by proposing three perspectives named Rational, Systemic, and Visual, and they were substantiated as the elemental units of differentiation and were demonstrated for their sufficiency in structuring such a knowledge base of conceptions.
It is important to note that, the three perspectives are not flat conceptions but rather structured and infinitely complex views that may sufficiently disclose the variants of any concept.
The philosophizing-capable machine would act on such variants-structured knowledge base using a monolithic philosophizing algorithm, which, although monolithic, is versatile and infinitely complex as well.
Consequently, both the variants-structured knowledge base of concepts and the philosophizing algorithm consume the philosophizing-capable machine, and we have to keep in mind that Philosophize-ability is devised as the mind’s capacity that underlies both the intentional and emotional mental productions. It substantiates analogy making/understanding, and whence, plays equivalently deterministic roles in art and science.
Consequently, both the variants-structured knowledge base of concepts and the philosophizing algorithm consume the philosophizing-capable machine, and we have to keep in mind that Philosophize-ability is devised as the mind’s capacity that underlies both the intentional and emotional mental productions. It substantiates analogy making/understanding, and whence, plays equivalently deterministic roles in art and science.
There are one of two paths the philosophizing algorithm may be applied over the knowledgebase of conceptions (see Figure 4):
1. The philosopher has to select specific path/s at a time (specific variants of the connected concepts), and this may be paralleled with the attentive system of any intelligent agent. In quantum mechanics, that may be metaphorically described as all the variants are in superpositions until observed by a philosopher, they have to collapse into specific states.
I need to remind the reader that when I mentioned in the introduction that DL’s limitations, although it is a universal function approximator, is inherently unrectifiable. That is because DL no may always act on specific paths rather than establishing a dialog with the superposition various paths, the way a philosopher does.
2. The other tactic is that the philosopher acts ontologically on the knowledge base itself (meta-philosophizing).
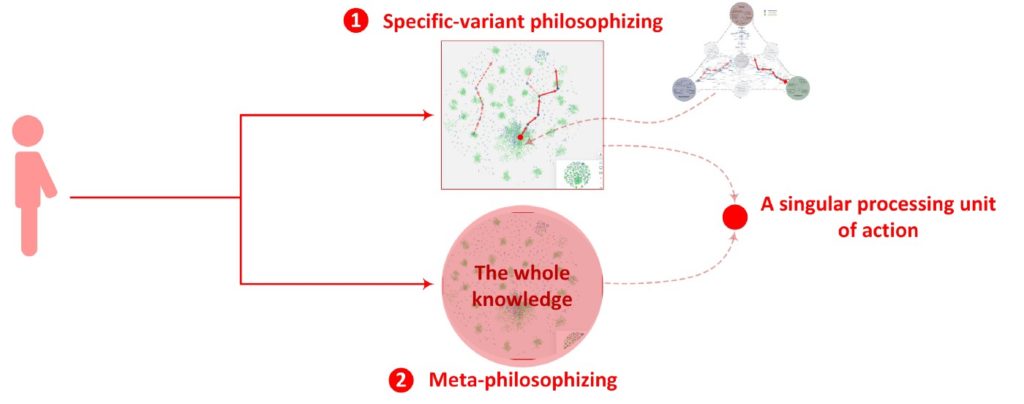
Figure 4 A philosophizing machine that either acts on specific variants of the concepts or on the whole knowledgebase. Nonetheless, it always approaches such a philosophizing procedure by a singular processing unit of action (Image by Author).
When the post assumes the concept-based approach to be the futuristic development of AI, it implies that any project should be involved in one of the previous two tactics. To prove the post’s POV, I nominated GLOM as a support of the first tactic’s specific variants path, DreamCoder (in my opinion) is a transition between the specifically selected variants and the supplementary of a fully-fledged knowledge base of concepts.
The project that I may be interested in the most is the OpenCog project that pursues structuring a knowledge base of atoms. Such a KB is assumed to be foundational for any AGI agent, and it is being experimented with over Sophia, the AGI robot star. Based on that I would compare the OpenCog’s Atomspace and my proposed concept-based knowledge base of conceptions.
Although It may be immature to pronounce specific results of the concept-based KB of conceptions before conducting the experimentations needed to ground the sufficiency/limitations of the three-perspectival constructed knowledge base of conceptions, I would merely utilize the theoretical discourse laid over the post as the basis for comparing between the two KBs.
The comparison between the two KBs is conducted over the two levels of the KB and the philosophizing algorithm (see Figure 5).
On the level of the declarative KB of concepts:
a) The concept-based KB is a Self-regulated declarative KB that encompasses the structured variants for any concept, and it initiates with minimal manual population.
b) The concept-based KB is defined and founded on analogical potentials. That is why it substantiates art and philosophizing behaviors by default.
c) The concepts in the concept-based KB are split into two levels; the first level is the fundamental concepts found in natural language while the second level introduces infinitely complex new concepts using the tools of iterative composition. Such dynamicity is less apparent in OpenCog.
d) The different faculties of the mid are self-regularly defined by the set of concepts that distinguish any of these faculties.
To align the terminology used by OpenCog and the Concept-based approaches, the philosophizing algorithm may be matched with the procedural memories devised by OpenCog, e.g., working memories and the other procedural ones. Based on that, the nest level of comparison is the philosophizing algorithm.
On the level of the philosophizing algorithm:
a) The concept-based philosophizing algorithm is a singular unit for processing that, although complex, may be defined by self-regulated procedures in contrast to the mostly manually curated OpenCog’s procedurality.
b) The concept-based philosophysing algorithm is Dynamic and may grow in complexity based on the concepts outlining the context of discourse, in contrast to the OpenCog’s defined procedurality as a priori.
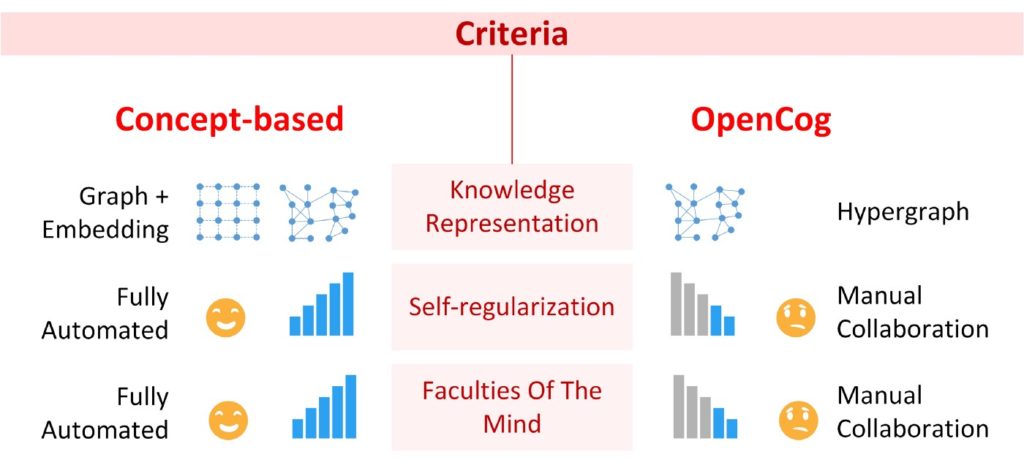
Figure 5 a comparison between the Concept-based and OpenCog approaches (Image by Author).
Conclusion:
AI is the science of exhibiting intelligent behaviors, which is implemented by mimicking intelligent systems. For example, deep learning is the field established by mimicking biological neural networks, and the post opens the door for considering the deep learning’s statistical mimicry of the concept-based paradigm as a trajectory of AI’s futuristic development. In fact, human intelligence has been theorized to be dependent on the fundamental building block of the concepts, and consequently, the AI goal of mimicking human intelligence may be fulfilled by mimicking the concept-based paradigm. This may be achieved qualitatively by either mimicking certain qualities of the concept-based paradigm or by supplementing knowledge bases of conceptions that sustain analogical capacities. Doing so infuses specific and general intelligence into a single realm that monolithically serves any task in hand (let that be conveniently referred to as specific or general).
I personally believe that this is the only way to resolve the brittleness and weaknesses experienced in contemporary narrow AI, and a fair part of the post was exerted to excerpt exemplar prevalent contemporary attempts as a support for the post’s point of view.
In the end, we have to admit that the development of the field of AI was historically tightly related to discoveries of other fields, e.g. the field of neurology, and synergizing the discoveries and findings of different fields would surely be a source of enrichment to the development of the field we all admire, the field of AI, and I have enclosed some of my research’s findings in support of that theoretical and empirical context.
This post is planned to be followed by a couple of more posts that explicate my Ph.D. project in greater detail. These serial posts are expected to end up by a universal conceptual space of the fundamental concepts of the language, such conceptual space is dually represented by embedding and graph-based representations, and both of these representations consume what is named as a “conceptualization space”. I would then, in these posts, demonstrate how a creative machine may manifest over such a conceptualization, and I may proclaim it to be a first of its kind. Additionally, I would debatably proclaim it to be a fundamental and a prerequisite knowledge base of any AGI agent, and consequently, general/specific-tasks intelligent agents are merely artificial intelligent agents, as debated in the post.
I hope you find the post informative, inspiring, and motivating. Thanks for reading my post, and until the next time.
References
[1] M. Mitchell, “Why AI is Harder Than We Think,” 2021.
[2] J. M. Bishop, “Artificial Intelligence is stupid and causal reasoning wont fix it,” 2020.
[3] M. Ezzat, “A Comprehensive Conceptualization of Urban Constructs as a Basis for Design Creativity: an ontological conception of urbanism for human-computer aided spatial experiential simulation and design creativity” , Springer, 2021.
[4] M. Ezzat, “A Comprehensive Proposition of Urbanism,” in New Metropolitan Perspectives, Springer, Cham, 2019.
[5] M. Ezzat, “A Triadic Model for a Comprehensive Understanding of Urbanism: With Its Potential Utilization on Analysing the Individualistic Urban Users’ Cognitive Systems,” LaborEst, vol. 16, pp. 25–31, 2018.
[6] G. Hinton, “How to represent part-whole hierarchies in a neural network,” 2021.
[7] A. Krizhevsky, I. Sutskever and G. E. Hinton, “ImageNet classification with deep convolutional neural networks,” in NIPS, 2012.
[8] K. Ellis, C. Wong, M. Nye, M. Sablé-Meyer, L. Cary, L. Morales, L. Hewitt, A. Solar-Lezama and J. B. Tenenbaum, “DreamCoder: Growing generalizable, interpretable knowledge with wake-sleep Bayesian program learning,” 2020.
[9] B. Goertzel, “The General Theory of General Intelligence: A Pragmatic Patternist Perspective,” 2021.
[10] “Open Cog,” [Online]. Available: https://opencog.org/.
[11] B. Goertzel, “CogPrime: An Integrative Architecture for Embodied Artificial General Intelligence,” 2012.
[12] “The Global AI Marketplace,” [Online]. Available: https://singularitynet.io/#.
[13] SingularityNET, “SingularityNET: A Decentralized, Open Market and Network for AIs (Whitepaper 2.0),” 2019.